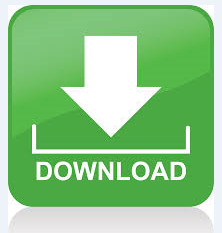
These narratives are largely variable between institutions and even healthcare professionals within them making it difficult for data extraction. In addition to structured data, a large amount of clinically relevant data remains present in an unstructured free-text format. The data within these records are expected to improve efficiency and overall healthcare. Nationwide adoption of Electronic Health Records (EHRs) has given rise to a large amount of digital health data, which can be used for secondary analysis. Our case study demonstrates the additional utility, of the features derived from RTF constructs, over plaintext oriented NLP.
#Trove free codes 2015 how to
We show how to parse these RTF constructs, analyze their prevalence and characteristics in the context of multiple types of EHR narratives.

We focus on the RTF constructs related to tables and sections that are not available in plain text EHR narratives. We investigate multiple types of EHR narratives in the Enterprise Data Warehouse from a multisite large healthcare chain consisting of both the academic medical center and community hospitals. Analysts who focus on structured EHR data often do not treat EHR narratives as structured NLP researchers often start from plain text instead of RTF files, casting structured data as narratives. There is rich structural information in these files which can be extracted and interpreted, yet such information is largely ignored. RTF is adopted by many medical information management systems.
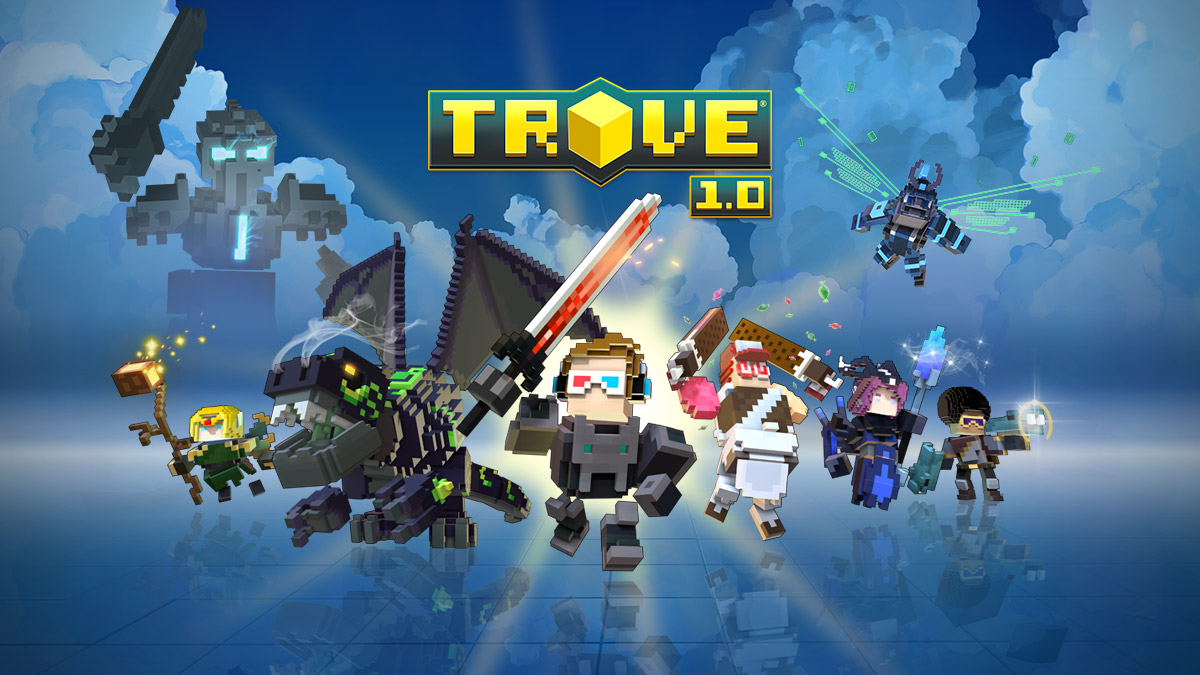
This study presents an approach for mining structured information from clinical narratives in Electronic Health Records (EHRs), by using Rich Text Formatted (RTF) records.
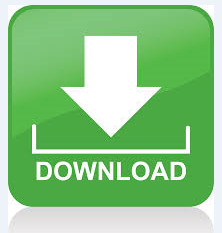